Abstract
Significant progress has been made towards mitigating climate change and its impacts across countries. However, the transboundary effect of CO2 emissions means that excluding the actions and inactions of certain countries and territories that escalate emissions is alarming. On this note, we examined the heterogeneous contribution of immediate and underlying drivers of emissions across 206 countries and territories for the period spanning 1960–2018. We deployed a dynamic panel estimation technique that accounts for cross-sectional dependence, heterogeneous parameters across countries, and dynamic correlated effects—a constraint for socio-economic, consumption- and pollution-based models. A global accounting of economic policy and debt, population structure, density and urbanization, and environmental-related aggregate indicators in a carbon emission function is presented. The empirical results demonstrate that the overarching effect of the instantaneous increase in economic development, population dynamics and energy utilization stimulate global emissions at national, urban and household levels across countries and territories. Industrialization and trade were found to escalate global pollution levels due to the impact of carbonized and energy-intensive economic structure in many developing and developed economies. Urbanization, urban income growth, and urban energy consumption are intertwined, hence, the institution of urban-related policy interventions is likely to negate the trio-impact on environmental sustainability. The triple effect (exploitation of natural resources, production and consumption) of economic development spurs environmental pollution, thus, calls for structural change from a carbonized to a decarbonized economy. The complex interaction highlights diversification of the energy mix by the inclusion of clean and renewable energy sources, fossil fuel-switching, and modern technologies like carbon capture and storage to improve energy efficiency and decline emission intensities.
Export citation and abstract BibTeX RIS

Original content from this work may be used under the terms of the Creative Commons Attribution 4.0 licence. Any further distribution of this work must maintain attribution to the author(s) and the title of the work, journal citation and DOI.
Introduction
The global debate on climate change and its impact has evolved within the past decades. Tackling climate change remains one of the difficult challenges in the 21st century. The visible signs of climate change events and hazards have accentuated the need to reduce anthropogenic greenhouse gas emissions from escalating. Such climate change-related events and hazards include several reported extreme temperatures [1, 2], flooding [3], drought [4], food insecurity [5], tropical cyclones [6], earthquakes, among others. Due to the 21st century styled economic pathway—carbon and energy-intensive based development, achieving the target of reducing the global ever-increasing average temperature to below 2 degrees Celsius appears difficult [7]. The situation is emboldened by the increasing population levels, reflecting on the exploitation of available natural resources to meet the growing demand while increasing waste generation. Aside from that, production and consumption remain the backbone of sustained economic development and a catalyst for anthropogenic emissions—a dilemma of achieving sustainable development goals. This has in effect triggered several climate change-related studies across different disciplines. Anthropogenic emissions, the principal factor of climate change, have several causes and dimensions, ranging from energy intensity [8], income level [9, 10], greenhouse gas intensity [11], population [12, 13], urbanization [14], governance [15], trade [16], technology [17], resource availability [18], industrialization [19], infrastructure [20], development [21], lifestyle and behaviour [22]. Dimensions of emissions range from economic incentives [23], non-climate policies [24], information provision [25], direct regulation [26], awareness creation [25], planning [27], research and development [28].
Several of these causes and dimensions have been used as indicators to assess the extent of emissions at national, urban or household levels—producing inconsistent results. For example, national-level data with annual periodicity were used to assess the urbanization and energy consumption attributable emissions in developing countries [29, 30] while urban level data were employed to examine climate change mitigation across urban areas in China [31] and the US [32]. The majority of studies [33–35] are mostly based on national-level data, with very little attention on either urban or household level—thus, policies are mostly based on a top-down approach. The inconsistency of results on emissions in previous studies can be attributed to sparsity and length of data, the use of indicators with heterogeneous characteristics across countries and estimation techniques utilized. Though some indicators with transboundary characteristics may require homogeneous parameter estimates, however, other indicators that are specific and vary between countries and territories require heterogeneous parameter estimation—a consideration that is omitted in extant literature.
Herein, comprehensively based consistent models that control for heterogeneity, cross-sectional dependence and common correlated effects across 206 countries and territories from 1960 to 2018 are constructed using the dynamic common correlated effects estimation technique. We follow both the United Nations Sustainable Development guidelines and Sustainable Development Goals to select important variables for national and household level accounting and construct urban level indicators. Our a priori expectations are based on the theoretical arguments that confirm a positive relationship between the environmental indicator and regressors. We construct choropleth maps using 59 year length of data to examine historical distribution across countries and territories.
The remaining sections of this paper are structured as follows: section 2 outlines the 'Materials & Methods' employed, section 3 presents the empirical results of the estimated models, section 4 provides a 'Discussion' of interpreted results and section 5 concludes the study by highlighting policy implications of the results and discussion.
Materials and methods
The study employed 206 countries and territories for the period spanning 1960–2018, with data series adapted from the World Bank development indicators [36]. Data from the World Bank ensure data quality and consistency across the selected countries. Data series include urban population (URBAN, number), Industry value-added (INDUST, constant 2010 US$), trade (TRADE, % of GDP), gross domestic product (GDP, constant 2010 US$), total population (POPU, number), energy consumption (ENGUSE, kg of oil equivalent per capita), per capita GDP (PGDP, constant 2010 US$), and carbon dioxide emissions (CO2KT, kt). GDP, income level, trade and industry value-added are useful indicators for economic policy and national debt accounting. Because the economic policy and national debt variables are measured in constant 2010 US$, fluctuation in prices across countries is expunged. While GDP is the aggregation of gross value added by indigenous producers and taxes on product excluding subsidies, income level accounts for GDP per midyear population [36]. Trade is the share of GDP that totals import and export of goods and services. It serves as an economic catalyst to either environmental pollution or sustainability [37]—depending on the type of goods and services imported and exported. Industry value-added encompasses value-addition in the manufacturing, construction, mining, water, electricity and gas sectors—without accounting for natural resources depletion and degradation [36]. Hereafter, Industry value-added was employed as a proxy for industrialization. Urban population accounts for people living in urban areas, hence, employed as an indicator for assessing urban sprawl. Population encompasses residents irrespective of citizenship or legal status. Population is a useful indicator for assessing the sustainability of available natural resources and social infrastructure [36]. Carbon dioxide is the main GHG that disturbs the radiative balance of the Earth and used as a reference gas for other greenhouse gases [38]. Carbon dioxide emissions account for CO2 from the production and consumption of fossil fuels and cement manufacturing—thus, CO2 was employed as a proxy for environmental pollution.
To examine the role of urban energy consumption, urban income level, energy intensity and per capita energy consumption on emissions, we constructed additional variables using the data from the World Bank. Due to the lack of data (urban energy consumption and urban income level) at the urban level for 206 countries and territories, the study used proportions assuming the total population corresponds to the aggregated GDP for a specific country. Country-specific GDP at the urban level can be calculated by plugging in the urban population, following a similar procedure used to develop sustainability index [39] and the Kaya identity [40]. The add-on variables were constructed using the following expressions:




where urban energy consumption (URBEN) was constructed by accounting for both urbanization (URBAN) and population in an energy consumption function. Generating urban income level (URBGD) was useful to account for urbanization and population in the era of economic development. Energy intensity (EINT) is a useful indicator for assessing the energy required per output, hence, useful for estimating energy inefficiency across economies. To construct a variable labelled as energy consumption per capita (PEINT), we estimated the share of energy per capita by controlling for population.
Panel data estimation
The study adopted panel data estimation techniques to account for the effect of urbanization, industrialization, energy utilization, trade and economic development on anthropogenic CO2 emissions. Panel data techniques estimate temporal inter- and intra- individual dynamics, and unobserved factors, hence, are more capable of modelling complex human behaviours compared to time series and cross-sectional settings [41]. Panel data encapsulate repeated observations of a group of individuals with both time series () and cross-sectional dimensions (
). The resulting models follow a standard methodological approach expressed as:

In equation (5), is the response variable with a vector of
control variables
resulting in the estimated parameters
....
and disturbances
, which are independent and identically distributed.
Equation (5) can be rewritten in a fixed-effects format expressed as:

In equation (6), represents the constant,
denotes the country-specific fixed effects—these are heterogeneous factors across cross-sectional units
but constant over time
—an unidentified parameter to be estimated. The fixed effects technique is useful in the presence of serial correction in the error term
The fixed effects technique assumes homoskedasticity in the estimation process, however, a departure from homoskedastic errors are eminent in pooled based panel data estimation, as such, a modified Wald test statistic for groupwise heteroskedasticity was employed as a postestimation technique for the fixed effects model.
Dynamic panel estimation
There are several panel data estimation techniques available in the existing literature, however, very few of the available techniques account for heterogeneous coefficients across different countries, cross-sectional dependence, and dynamic correlated effects—a requirement for most socio-economic, consumption and pollution models. Hence, appropriate for this study in the attempt to account for the heterogeneous effect of urban sprawl, industrialization, trade and economic development on environmental pollution. The novel dynamic common correlated effects [42] technique is essential for panel data with large cross-sectional units and time periods, a situation evident in this study—∼206 countries and territories, 59 time periods (1960–2018) and ∼9849 observations.
The advantages of the dynamic common correlated effects outweigh both mean group and pooled mean group estimators. Apart from the dynamic common correlated effects specification, first, it estimates both homogeneous and heterogeneous parameters and allows for endogenous regressors. Second, it computes the cross-sectional dependence test and corrects small sample time series bias [42]. Third, it accommodates both balanced and unbalanced panel data setting. The econometric model for dynamic panel estimation that controls for heterogeneity can be expressed as:
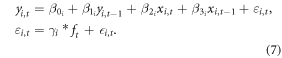
In equation (7), the response variable of the cross-sectional units
in periods
is regressed on the control variables
—assumed to be strictly exogeneous, resulting in the estimated heterogeneous coefficients
's—randomly distributed around a common mean and i.i.d. errors
with unobserved common
and heterogeneous factor
loadings.
The dynamic common correlated effect is the empirical specification of equation (7) without lagged independent variable expressed as:
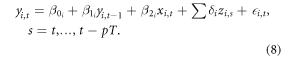
In equation (8), the number of lags of the cross-sectional means
(partialled out) are incorporated in the dynamic panel data estimation model to achieve consistency [43].
Panel estimation process
Prior to the estimation of the dynamic panel common correlated effects, the study applied panel fixed-effects regression model with modified Wald test to ascertain whether estimated coefficients are homogeneous or heterogeneous. The modified Wald test examines the groupwise heteroskedasticity in the fixed-effects model which assumes homoskedasticity—but is often violated due to specific error variances of cross-sectional units. The application of the modified Wald test [44] stems from the results of the Jarque–Bera test statistic—which confirms the data series violates the assumption of normality (see table S1, available online at stacks.iop.org/ERL/15/034049/mmedia). Significantly, the modified Wald test statistic accommodates panel settings with unequal distribution of observations across cross-sectional units—a typical case in this study. The null hypothesis of homoskedasticity is rejected for the alternative—heteroskedasticity—if the probability value of the Chi-square from the Wald test estimation results is less than the 5% significance level. Heteroskedasticity leads to inconsistent and bias coefficient estimates in traditional techniques. Hence, testing for heteroskedasticity among estimated coefficients from a model is useful in the selection of an appropriate dynamic estimation technique capable of confronting panel heterogeneity and cross-sectional dependence (see supplementary text).
Results
Figure 1 depicts the descriptive geographic mean distribution of urban income level (URBGD), urban population (URBAN), CO2 emissions (CO2KT), energy use (ENGUSE), GDP, Industry value-added (INDUST), GDP per capita (PGDP), trade, total population (POPU) and urban energy consumption (URBEN).
Figure 1. Geographic mean distribution of (a) Urban income level [constant 2010 US$] (b) Urban population [Number] (c) CO2 emissions [kt] (d) Energy use [kg of oil equivalent per capita] (e) GDP [constant 2010 US$] (f) industry value-added [constant 2010 US$] (g) GDP per capita [constant 2010 US$] (h) Trade [% of GDP] (i) total population [Number] (j) Urban energy consumption [kg of oil equivalent per capita].
Download figure:
Standard image High-resolution imageFrom the choropleth map in figure 1(a), (b), the mean distribution of URBGD is high in the US (∼7.4 Trillion) followed by Japan (∼3.2 Trillion, constant 2010 US$), Germany (∼2.0 Trillion, constant 2010 US$), France (∼1.4 Trillion, constant 2010 US$), United Kingdom (∼1.3 Trillion, constant 2010 US$), China (∼1.1 Trillion, constant 2010 US$), Italy (∼1 Trillion, constant 2010 US$), Russia (∼957 Billion, constant 2010 US$), Brazil (∼952 Billion, constant 2010 US$), and Canada (∼812 Billion, constant 2010 US$). URBGD is low in Africa, Greenland, Denmark, Russia and some Asian countries. Even though URBGD is high in the US, urban population is high in China (∼336 Million), India (∼233 Million) and the US (∼194 Million), respectively. The URBAN levels are moderate in Germany, France, Russia, United Kingdom, Mexico, Brazil, and some Asian countries. Globally, the average urban population is relatively low in most African countries.
The average distribution of CO2KT and ENGUSE in the choropleth maps presented in figures 1(c), (d) shows the USA and China as the major contributor of carbon emissions—although carbon emissions in the US (∼4.7 Million kt) are higher compared to China (∼3.1 Million kt). CO2KT is on the rise in Russia, India and Germany. Although CO2KT is high in India, its ENGUSE is low. In Africa, South Africa, and Libya consume a lot of energy compared to other African countries. The US, Iceland and Canada are countries in this study that consume lots of energy.
The US has the highest GDP within the study period followed by China, the United Kingdom, France, Germany, Russia, and Brazil. The countries within the African continent have the lowest GDP compared to the global average in the study period. The US remains the highest in terms of industry value-added. The mean distribution of industry value-added reveals China and Germany as countries with high industrial activities after the US, followed closely by Canada, Russia, Brazil, France and UK, respectively but low in Denmark and Africa (see the choropleth map in figure 1(e), (f)).
Norway has the highest average per capita income for the study period, followed closely by the US, Canada, Denmark, Australia, Sweden, Finland, Spain, Germany, Saudi Arabia and Greenland. The contribution of trade to GDP is relatively high in Africa contrary to the USA (see figure 1(g), (h)).
China has the highest mean distribution of the total population (from the study choropleth map in figure 1(i), (j)). The total population in India, Nigeria, Russia, Pakistan, the US and Brazil are considerably high while urban energy consumption is high in Iceland. The US, Canada, Australia, Russia, Saudi Arabia, UK, France, and Germany are countries in this study that consume lots of energy in their urban cities. Generally, countries in Africa have the lowest average URBEN. China has the largest population yet consumes less energy in urban areas, however, Iceland with a smaller population is connected with higher energy consumption in urban areas.
The initial estimation strategy adopted examines the country-specific fixed-effect by assuming model homoskedasticity. To test the validity of the assumption, the study employed the modified Wald statistic for country-specific heteroskedasticity in the fixed-effect model. The resulting estimated parameters based on 11 models are presented in table 1. According to the Intergovernmental Panel on Climate Change (IPCC) fifth assessment report, energy consumption, economic development, population, and GHG intensity represent the immediate drivers of anthropogenic emissions [45]. Based on this assertion, three of the four drivers due to data availability were tested in model 1. The output reveals that 166 of 206 countries and territories with 5366 observations qualified for the assessment. The model was statistically significant at 1% level (p-value < 0.01), with 90% predictive power (R2 = 0.90). All the coefficients on the estimated parameters excluding the constant shows a positive relationship with CO2 emissions. In the order of intensity, population, energy consumption, and economic development exacerbate emissions in at least 166 countries and territories—consistent with the IPCC report. The role of rural-urban migration, intensive energy utilization and increasing levels of income cannot be ignored in this 21st century of climate change mitigation. Model 2 examined how an economy based on energy inefficiency (energy intensity), urban sprawl and income impact emission levels—by controlling for 166 country-specific fixed-effects based on 5366 observations and 82% predictive power. Evidence shows the sign on the coefficients is positive and statistically significant (p-value < 0.01). The magnitude of the effect follows as 1.05%, 0.75% and 0.51% for a percentage increase in urbanization, income level, and energy intensity, respectively. In models 3–5, underlying drivers like trade and industrialization plus consumption per capita, population, economic growth, and urbanization were examined in a carbon function across ∼179 countries and territories with ∼5585 observations at ∼91% predictive power. Though the sign on the estimated parameters is positive, all the indicators are statistically significant (p-value < 0.01) in the function except trade. This indicates that while the effect of trade is insignificant, rural-urban migration, energy consumption per capita, economic growth, population, and intensive industrialization spur emissions. All the estimated models thus far concentrated on the national level data, however, in model 6 growth in income and energy consumption at the urban level were estimated in a carbon function to purposely ascertain the extent of the indicators on emissions. The study confirms a statistically significant positive effect of urban energy consumption and urban income level on emissions. The empirical results highlight the intensifying effect of energy consumption and income level in both national and urban level. In model 7, the output confirms previous models except for trade which turns significant. In models 8–11, the individual effect of aggregate energy consumption, industrialization, population, and urban energy consumption confirm the previously estimated models in almost 206 countries and territories. The modified Wald test statistic for all the estimated fixed-effect regression models reject the null hypothesis of homoskedasticity at 1% significance level—indicating a deviation from homoskedastic errors and a violation of normality assumption.
Table 1. A fixed-effect regression model with modified Wald test for groupwise heteroskedasticity.
lnCO2KT | Model 1 | Model 2 | Model 3 | Model 4 | Model 5 | Model 6 | Model 7 | Model 8 | Model 9 | Model 10 | Model 11 |
---|---|---|---|---|---|---|---|---|---|---|---|
lnENGUSE | 0.57* [0.02] | — | — | — | — | — | 0.67*[0.01] | 1.18*[0.01] | — | — | — |
lnEINT | — | 0.51*[0.01] | — | — | — | — | — | — | — | — | — |
lnPEINT | — | — | 0.52* [0.02] | — | — | — | — | — | — | — | — |
lnGDP | 0.31*[0.01] | — | — | — | 0.34* [0.03] | — | — | — | — | — | — |
lnPGDP | — | 0.75*[0.01] | — | — | — | — | — | — | — | — | — |
lnINDUST | — | — | 0.24*[0.01] | 086*[0.01] | 0.30*[0.02] | — | — | — | 0.85*[0.01] | — | — |
lnTRADE | — | — | 0.01[0.01] | 0.00[0.01] | 0.01[0.01] | — | 0.08*[0.01] | — | — | — | — |
lnPOPU | 0.67*[0.02] | — | — | — | 0.76*[0.03] | — | — | — | — | 1.76*[0.01] | — |
lnURBAN | — | 1.05*[0.01] | 0.98*[0.02] | — | — | — | 0.76*[0.01] | — | — | — | — |
lnURBEN | — | — | — | — | — | 0.39*[0.01] | — | — | — | — | 1.02*[0.01] |
lnURBGD | — | — | — | — | — | 0.52*[0.01] | — | — | — | — | — |
CONS | −12.21*[0.22] | −3.64*[0.70] | −6.17*[0.21] | −10.25*[0.17] | −17.94*[10.41] | −2.77*[1.24] | −6.78*[0.14] | 1.60*[0.11] | −10.02*[0.16] | −17.94*[0.22] | 3.38*[0.06] |
Diagnostics | |||||||||||
Obs | 5366 | 5366 | 4026 | 5585 | 5578 | 5366 | 5173 | 5820 | 5946 | 9849 | 5820 |
Groups | 166 | 166 | 157 | 179 | 179 | 166 | 164 | 172 | 187 | 206 | 172 |
Prob > F | 0.00* | 0.00* | 0.00* | 0.00* | 0.00* | 0.00* | 0.00* | 0.00* | 0.00* | 0.00* | 0.00* |
R-squared | 0.90 | 0.82 | 0.89 | 0.91 | 0.85 | 0.67 | 0.93 | 0.25 | 0.91 | 0.60 | 0.25 |
Prob>![]() |
0.00* | 0.00* | 0.00* | 0.00* | 0.00* | 0.00* | 0.00* | 0.00* | 0.00* | 0.00* | 0.00* |
aRepresents the Modified Wald test for examining groupwise heteroskedasticity in the fixed-effect regression model.
The diagnostic test underscores the need to validate the fixed-effects approach by re-estimating the models using a technique that controls for dynamic common correlated effects in the presence of heterogeneity. Table 2 presents the empirical results of the panel dynamic common correlated effects. All the models are statistically significant at p-value < 0.01 with predictive power ranging from 26% to 60%. The coefficient of the 1 year-lagged emissions () in all the models is negative and statistically significant at 1% level. Indicating that the global emissions of carbon dioxide is a dynamic common correlated process. Though there exist differences in the magnitude of the estimated parameters compared to the fixed-effects model, however, the sign of both models is consistent, confirming our a priori expectations. To test the consistency of the dynamic common correlated effects estimator, we employed Pesaran's cross-sectional dependence test (CD Statistic) to examine the independence of the residuals under the null hypothesis of weakly cross-sectional dependent error terms. The CD test Statistics in table 2 reveals that the probability of the estimates is greater than the 5% significance level, hence, cannot reject the null hypothesis—declaring that the estimated parameters are consistent to make unbiased statistically inferences.
Table 2. Panel dynamic common correlated effects.
lnCO2KT | Model 1 | Model 2 | Model 3 | Model 4 | Model 5 | Model 6 | Model 7 | Model 8 | Model 9 | Model 10 | Model 11 |
---|---|---|---|---|---|---|---|---|---|---|---|
![]() |
−0.79*[0.02] | −0.76*[0.02] | −0.83*[0.03] | −0.54*[0.03] | −0.74*[0.03] | −0.69*[0.02] | −0.75*[0.02] | −0.52*[0.02] | −0.50*[0.03] | −0.36*[0.02] | −0.53*[0.02] |
lnENGUSE | 1.04*[0.18] | — | — | — | — | — | 0.88*[0.08] | 0.80*[0.13] | — | — | — |
lnEINT | — | 0.84*[0.07] | — | — | — | — | — | — | — | — | — |
lnPEINT | — | — | 0.88*[0.14] | — | — | — | — | — | — | — | — |
lnGDP | 0.19*[0.06] | — | — | — | 0.26**[0.14] | — | — | — | — | — | — |
lnPGDP | — | 1.08*[0.08] | — | — | — | — | — | — | — | — | — |
lnINDUST | — | — | 0.15*[0.05] | 0.37*[0.04] | 0.24*[0.07] | — | — | — | 0.34*[0.04] | — | — |
lnTRADE | — | — | 0.04[0.03] | 0.03[0.03] | 0.05[0.03] | — | 0.04*[0.02] | — | — | — | — |
lnPOPU | 0.73*[0.20] | — | — | — | 0.95**[0.49] | — | — | — | — | 1.13**[0.66] | — |
lnURBAN | — | 0.96*[0.17] | 0.72[0.45] | — | — | — | 0.64*[0.20] | — | — | — | — |
lnURBEN | — | — | — | — | — | 0.64*[0.05] | — | — | — | — | 0.60*[0.04] |
lnURBGD | — | — | — | — | — | 0.15*[0.05] | — | — | — | — | — |
CONS | −9.74**[4.16] | 2.44[2.99] | −3.33[11.69] | 1.70[2.59] | −3.31[10.41] | 1.94[1.24] | −5.38[4.72] | −3.62*[0.98] | −3.83[3.04] | 1.24[3.99] | −0.48[0.44] |
Diagnostics | |||||||||||
Obs | 5167 | 5167 | 3791 | 5451 | 5322 | 5167 | 4977 | 5616 | 5872 | 9635 | 5616 |
Groups | 134 | 134 | 114 | 161 | 149 | 134 | 131 | 139 | 177 | 203 | 139 |
Prob > F | 0.00* | 0.00* | 0.00* | 0.00* | 0.00* | 0.00* | 0.00* | 0.00* | 0.00* | 0.00* | 0.00* |
R-squared | 0.59 | 0.59 | 0.60 | 0.39 | 0.50 | 0.53 | 0.56 | 0.46 | 0.33 | 0.26 | 0.46 |
Root MSE | 0.09 | 0.09 | 0.09 | 0.12 | 0.12 | 0.10 | 0.10 | 0.11 | 0.13 | 0.17 | 0.11 |
CD Statistic | −0.37 | −0.93 | −1.44 | 0.20 | 0.25 | −0.80 | −0.69 | 0.15 | −0.63 | 0.79 | 0.03 |
p-value | 0.71 | 0.35 | 0.15 | 0.84 | 0.80 | 0.42 | 0.49 | 0.88 | 0.53 | 0.43 | 0.97 |
Notes: denotes convergence of CO2 emissions across countries.
Discussion
Evidence from the empirical analysis shows that most of the countries and territories under review exhibit the tendency of achieving convergence in emissions. However, this study cannot confirm the validity of either an absolute or conditional convergence [46]. This means that there may be two possible interpretations of this outcome in terms of policy perspective. First, there is a tendency that the dynamic common correlated effects lead to a single equilibrium (absolute convergence). If this occurs, then the disparity in emissions between low-, middle- and higher-income countries and territories is likely to unite. Alternatively, if country-specific equilibrium (conditional convergence) occurs, then the disparity in emissions between countries of different income and emission levels can be persistent. Thus, lower emission countries, specifically, countries in Africa with lower economic output cannot catch-up with the high levels of emission in developed countries. In both situations, because the coefficient on the lagged CO2 emissions is negative and significant in all models, lower-CO2 emitting countries with small levels of emissions will grow with a higher growth rate.
From a policy perspective, the results have policy implications for population, urban and household levels. At the population level, increasing the three immediate drivers of anthropogenic emissions—namely population, economic growth and energy consumption/intensity negates environmental sustainability by spurring CO2 emissions. The magnitude of these effects on emissions may differ across countries and territories depending on the size of its population, economic structure, elements of the energy mix or energy required per economic growth [13]. Increasing population triggers demand for food, water, energy, and its related service, land, goods and services, hence, pressures available natural resources by reducing the regenerative capacity. Several studies have demonstrated the positive impact of population on historical trend and expected future emissions [13, 47]. It appears that slower population growth spurs economic development which in turn drives up emissions, however, the impact is often not huge enough to negate environmental sustainability [48]. Instantaneous CO2 emissions are triggered when the economic structure is carbon-intensive and relies on mainly fossil fuel energy sources to produce goods and services. This notion might not always be the case across countries and territories per the environmental Kuznets curve hypothesis. The economic structure namely agrarian, industrial and services determine the magnitude effect of economic development on emissions. Least, low to lower-middle-income countries are typically inclined to agrarian-based economy, where the level of emissions tends to increase with increasing economic activities. Emissions at this economic structure tend to escalate at a faster pace with vintage technologies at pre-harvesting, harvesting and post-harvesting, hazardous waste generation and exploitation of reserves and protected areas [49]. In the industrialized economy, typically upper middle income and above, there is a dominance of carbon and energy-intensive manufacturing industries. The composition of this economic structure often drives emissions compared to agrarian-based emissions due to the dependence on fossil fuels and carbonized activities to produce competitive goods and services. This explanation underpins why anthropogenic emissions exacerbate with increasing levels of industrialization [50]. Apart from industrialization, other underlying drivers of emissions in this study include trade and urbanization. Trade is observed as a partnership between countries and territories but can often be a channel to transfer pollution across boundaries. Countries with stringent environmental regulations often transfer their polluting technologies, carbonized and energy-intensive industries to jurisdictions with laxed environmental regulations to haven pollution [51]. This justifies why pollution was found to worsen with expanding trade across countries and territories.
At the urban level, increasing trends of rural-urban migration, urban income growth, and urban energy consumption escalate emissions. While several studies found similar results, inter alia, in China [14], and Europe [12], other few extant literature are inconsistent with the outcome. High urban population growth drives emissions by increasing energy demand and supply, pressures social amenities, affects urban concentration levels, and triggers socio-economic challenges. Growth in urban population increases urban transportation (growth in motor vehicles), which directly spur energy consumption, especially from oil and gas [52]. This growth cycle between urban sprawl and urban transportation increases both ecological and carbon footprint, hence, escalating emissions. The transportation sector is estimated to contribute directly to greenhouse gas emissions by 14%—with its major share from urban areas [45]. Though at the initial stages, urban sprawl exacerbates emissions—it is anticipated that urban population will mostly adopt sophisticated and modern technologies at higher income levels that would in the long-term offset environmental quality, however, the magnitude of this effect depends on the willingness to pay for a clean environment.
Accounting for environmental pollution at the household level is critical for awareness creation and an early warning sign of climate-related risk. The empirical results revealed that expanding income level and energy consumption per capita escalate emission levels. Meaning that controlling for population dynamics in economic growth and energy consumption is useful for understanding the contribution of the household as an element for curbing emissions. This outcome is consistent with the IPCC fifth assessment report on drivers of climate change [45]—indicating that integrating climate change awareness and adaptation option using the bottom-up approach (i.e. from household to the national level) of policy formulation is worthwhile.
Conclusion
Though efforts have been made in different countries to improve energy efficiency, however, our study demonstrated that economic development is powered by high levels of energy consumption—which in effect spurs emissions. Meaning that high energy intensity hampers energy efficiency and negates environmental sustainability. Population dynamics play a critical role in climate change mitigation, especially in terms of lifestyle and behavioural patterns. For example, achieving energy efficiency begins at the household level through an attitudinal change such as willingness to pay and adopt energy conservation and management options. Thus, pollution abatement begins with the minimization of households' carbon footprint. Slower population growth is expected to decline the sensitivity and vulnerability of climate change by reducing environmental pressures on natural resources. This in effect increases climate change adaptation by increasing the biocapacity of available resources to regenerate itself. The adverse effect of population growth and urban sprawl burdens ecosystem services and natural resources—leading to the ecological deficit and increase the population's exposure to climate change variability. Because the emission of CO2 is transboundary and not localized, there is a possibility of spillover and synergy between countries, hence, global policies on emissions across countries and territories would be useful in mitigating global emissions. From a policy perspective, our study showed that emission reduction is a collective effort from the household level to urban settings and the national level. Thus, expanding household income levels and reducing inequality will improve lifestyle and behaviour towards climate change reduction at the household level. Urban areas serve as a haven for industrial and economic expansion, hence, characterized by high energy demand and consumption, population growth and high levels of emissions, leading to high climate risks. Implying that, infrastructural development (transport, housing, water) and sustainable urban planning are useful policies that reduce climate risks and enhance a sustainable urban environment. National policies that promote energy conservation and management options and improve the economic structure from energy and carbon-intensive economy to a decarbonized economy are essential to mitigating climate change and its impact.
Data availability
The data that support the findings of this study are available from the corresponding author upon reasonable request.